Unlocking AI in Highly Demanding Chip Manufacturing Environments
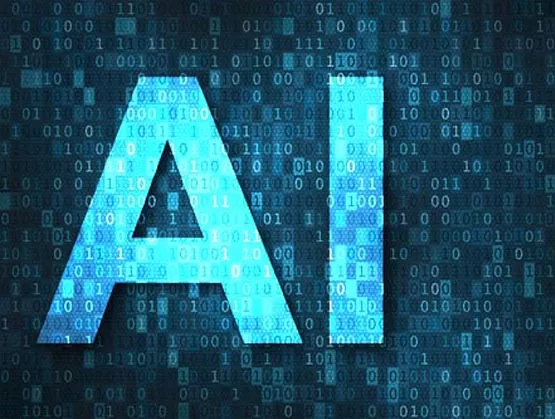
Despite its great promise to help streamline semiconductor manufacturing, artificial intelligence (AI) has largely failed to deliver on its potential despite significant investments in data infrastructure and talent. Understanding the reasons behind this failure is a critical step towards unlocking AI and associated ROI.
Empirical evidence points to four main reasons for failure:
- Insufficient model robustness
- Limited actionability
- Unreliable infrastructure
- Inefficient team organization
Strengthening AI models
Manufacturing data is extremely dynamic and noisy, which makes the development of robust predictive models challenging. To be considered robust in manufacturing environments, an AI model needs to deliver stable predictive performance on limited exploitable data, across multiple product types and maintain performances over time with minimal retraining frequency.
The first step to training any predictive model is selecting the right input variables. Relying purely on data can be deceptive: a traditional data-based feature importance analysis would not pick up variables that remain stable over time, yet a sudden change in those normally stable variables is very likely to seriously disrupt industrial processes, making the validation of variable selection necessary.
Once variables have been selected, the next step is to distinguish noise from informative signals. Capturing the data that accurately reflects processing conditions and not peripheral phenomena such as machine ramp-up, cool down or cleaning is essential.
Lastly, the model’s predictive accuracy needs to be assessed relevantly. A relevant assessment implies two critical steps:
- Simulating production conditions and especially the time sequence of events: testing the model on a randomly extracted set of data means “future” data can be given to the model for training which gives an artificial boost to predictive accuracy.
- Averaging the error over the entire test set is also deceptive as it fails to capture the model’s ability to predict extreme events – such as out of spec values – which is precisely what it is meant to do.
Confirming performances in production conditions and on extreme events ensures that a model is truly predictive and ready to be deployed.
Improving actionability
Once the model’s performance is confirmed, it’s important to make sure it can support production decisions by first understanding the user. Manufacturing engineers spend a large amount of their time solving problems using contextual evidence to understand what went wrong and how to fix it.
If they are to adopt AI and rely on predictions to make those production decisions (Should I stop the machine? Should I update some parameters?), they need context.
Understanding additional context that is needed entails addressing questions raised by engineers when a model raises an alert:
- Is the alert important?
- Which essentially means is this alert real?
- Disrupting a high-volume production line will impact output and is therefore comes at a high cost.
- It is therefore critical to indicate if a prediction is reliable or not, which for AI means confirming the model is predicting based on data it has already been trained on.
- What is the source of the alert?
- The first step to resolving an issue is identifying where this issue is coming from. This traditionally implies extensive data analysis under huge time pressure.
- Correlation is not causation but highlighting the most important deviations in input data helps point engineers in the right direction and enables significant time savings.
- What should I do?
- Engineers rely on pre-determined action plans (or OCAPs – out of control action plans to implement corrective actions).
- Aligning the output of a predictive model with existing OCAPs helps maximize continuity in the engineer’s workflow, which is key to adoption.
- It is also necessary to revisit OCAPs to fully integrate AI in the resolution process – this means standardizing the way engineers interpret what the model is telling them.
AI can be an extremely powerful tool for manufacturing engineers, but in order to fully support them it needs to be contextualized. Actionability is the number one driver of adoption and traditional black-box approaches fail to adapt to the constraints and challenges associated with managing manufacturing processes.
Increasing infrastructure reliability
While establishing a reliable model able to support the engineer in managing the production process is an achievement in itself, the next step – getting the model to work – is crucial. In other words, the model needs to be reliably fed with the data it requires to run.
Getting the right data, in the right format, at the right place and at the right time is extremely difficult, especially when facing:
- Stringent network and security constraints: Semiconductor fabs are often not even connected to the internet.
- Multiple legacy systems layered on top of each other and communicating sometimes inefficiently: This is particularly true in industry that have experienced high consolidation, such as semiconductor manufacturing.
- Fragmented data sources: Sensor data, results quality tests and maintenance logs are often collected and stored in different databases, which can be managed or updated differently.
There is no silver bullet to maintaining a fully functional AI infrastructure in this context, but four guiding principles can greatly improve the availability of the entire predictive system:
- Involve IT early: Involving IT departments is often associated with heavy qualification processes and administrative delays, and therefore often done once the proof of concept has been made. This is a mistake as compatibility with existing systems is a critical element of feasibility.
Keep it simple: In order to cut corners or to make the most out of past investments, it can be tempting to use multiple existing softwares or tools as building blocks for the entire pipeline. While it makes theoretical sense, it often multiplies the complexity of the system and makes it more difficult to support. The more direct the connection between the data and the model the better.
- Build gates: Integrating clear gates and handshakes at the intersection of the different elements of the pipeline enables more granular monitoring of the pipeline and a clearer escalation plan (provided that ownership is clearly established for each segment of the pipeline).
- Redundancy is paramount: Assume the worst and build in double or even triple redundancy for all critical parts of the system (data, infra, software). It can come as a trade-off, implying less but more robust capabilities.
Maintaining a robust data pipeline in manufacturing environments requires team coordination, robust design and a clear understanding of pre-existing systems. It can be tempting to build sophisticated state-of-the-art systems, but once you are in production, any downtime will impact the performance of the factory – so failure is not an option!
Streamlining team organization
While AI is often described as potentially transformative by CEOs, the way AI projects are handled in big manufacturing companies can be self-defeating. Many AI initiatives across a broad spectrum of use cases never make it from projects to products. One reason is the poor use of talent and skills due to inefficient team organizations.
There are two main ways AI projects are managed:
- Central AI teams
A small group of data scientists working from HQ on the company’s digital transformation often serve as internal data consultants pulled in every direction by different departments.
- The teams are often too distant from real-life operations to build an actionable project and lack the skills to deploy and maintain models (data scientists are not ML or DevOps engineers).
- Individual engineers
- Each engineer typically oversees a single, “local” use case or process step.
- These side projects often lack resources and visibility necessary to scale.
While team and individual initiatives can yield interesting results, many projects are insufficiently packaged and do not constitute a management-ready business case able to support the allocation of the resources necessary to drive large-scale implementation and impact.
Building the business case requires a cross-functional effort that can be coordinated as follows:
- Manufacturing engineer: Define and implement new workflow including AI, in essence validating adoption of the tool.
- Engineering manager: Build scaling plan and secure engineering time necessary for implementation.
- Data scientist: Validate model methodology and results.
- IT engineer: Validate compatibility with existing systems and plan pipeline maintenance
- Quality engineer: Perform comprehensive risk assessment.
- Industrial engineering: Quantify impact on key manufacturing indicators and associated value.
Combining all of these elements provides a complete picture of how the proposed AI solution could be used, valued, implemented and scaled and can be sufficient in supporting a Go/No go decision from management for deployment in production.
SEMI Smart Manufacturing Initiative Facilitates Adoption of AI
Successfully deploying AI in a high-value manufacturing environment, such as a semiconductor fab, by focusing on predictive performance alone is not enough. Building a comprehensive business case evidencing the expected impact on manufacturing performance, the robustness of the system and the feasibility of scaling is a critical and often neglected step.
In order to help define and spread those best practices throughout the industry, Lynceus partners with the SEMI Smart Manufacturing Initiative. Our common goal is to facilitate the adoption of AI in semiconductor manufacturing through the development of actionable tools and guidelines, designed to help those in the front lines of production take informed decisions.
The good news is that there is no scientific barrier to making AI work. AI needs to adapt to the organization and operations it seeks to impact.
About the Author
David Meyer founded Lynceus with Guglielmo Montone in 2020, with the ambition to build a comprehensive operating system for high value manufacturing. David focuses on assembling a world class team and gathering the resources necessary to support the Lynceus vision and effectively deploy AI in today’s most challenging industrial environments. Prior to Lynceus, David held various Ops leadership positions in companies like Uber and Circ (acquired by Bird), and advised investment funds on the acquisition of industrial companies. David holds an MSc from ESSEC Business School (France).